Optical tissue clearing and machine learning can precisely characterize extravasation and blood vessel architecture in brain tumors
Precise methods for quantifying drug accumulation in brain tissue are currently very limited, challenging the development of new therapeutics for brain disorders. Transcardial perfusion is instrumental for removing the intravascular fraction of an injected compound, thereby allowing for ex vivo assessment of extravasation into the brain. However, pathological remodeling of tissue microenvironment can affect the efficiency of transcardial perfusion, which has been largely overlooked.
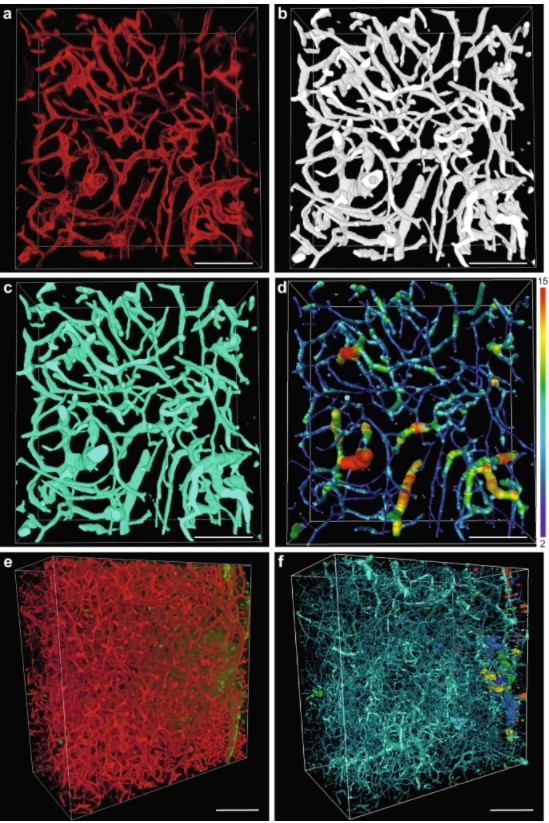
We show that, in contrast to healthy vasculature, transcardial perfusion cannot remove an injected compound from the tumor vasculature to a sufficient extent leading to considerable overestimation of compound extravasation. We demonstrate that 3D deep imaging of optically cleared tumor samples overcomes this limitation. We developed two machine learning-based semi-automated image analysis workflows, which provide detailed quantitative characterization of compound extravasation patterns as well as tumor angioarchitecture in large three-dimensional datasets from optically cleared samples. This methodology provides a precise and comprehensive analysis of extravasation in brain tumors and allows for correlation of extravasation patterns with specific features of the heterogeneous brain tumor vasculature.